Unearthing the Past: Future Technologies for Translating Ancient Languages
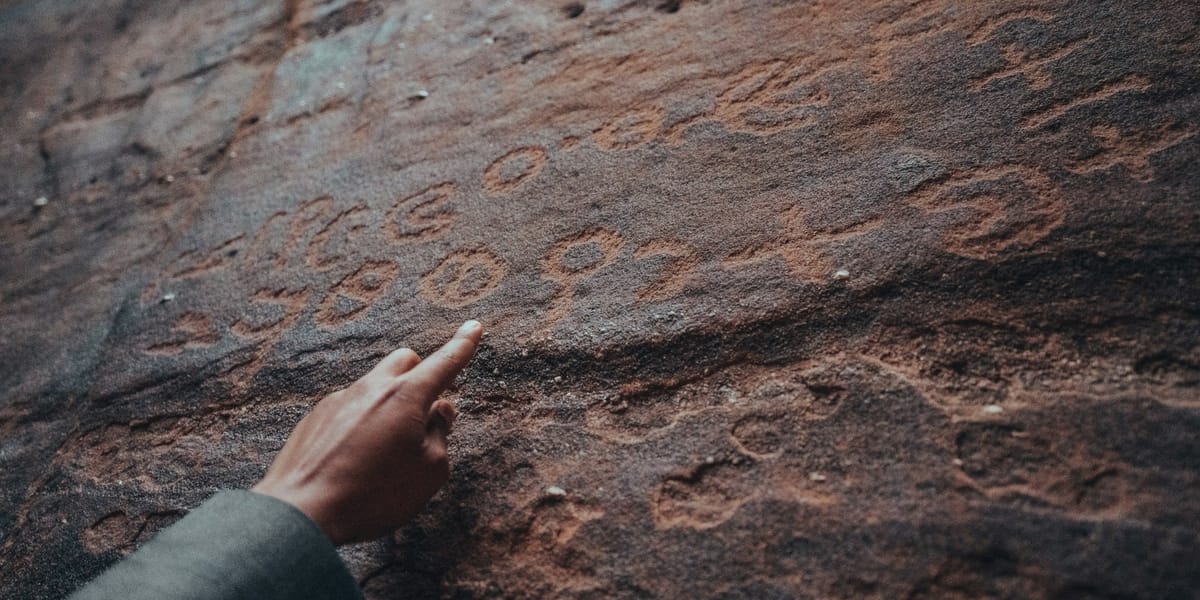
Ancient languages hold the key to unlocking the secrets of bygone civilizations, offering invaluable insights into history, culture, and philosophy. However, deciphering these "dead" languages often proves a laborious task, reliant on the expertise of a dwindling pool of linguists. Fortunately, the future holds promise with advancements in technology poised to revolutionize the field of ancient language translation.
One promising avenue lies in the continued development of Artificial Neural Networks (ANNs). These complex algorithms, inspired by the structure of the human brain, can learn intricate patterns within large datasets of text. Pioneering research by Zhiqi Shen et al. (2018) employed an ANN to translate Chinese characters from oracle bone inscriptions, some of the earliest forms of Chinese writing. The model achieved impressive results, suggesting the potential of ANNs to bridge the vast gap between ancient and modern languages.
Machine Learning (ML) algorithms are another powerful tool for deciphering ancient languages. By analyzing vast quantities of bilingual texts, where an ancient language appears alongside its modern equivalent, ML models can learn the underlying grammatical structures and vocabulary correspondences. One such example is the work of Natalia Gridina et al. (2020) who developed an ML model capable of translating Akkadian, an ancient Mesopotamian language. Their model achieved significant accuracy, paving the way for the automated translation of a wealth of historical texts.
Beyond mere translation, future technologies may even assist in the reconstruction of lost languages. This ambitious goal hinges on the development of advanced Natural Language Processing (NLP) techniques. NLP algorithms can analyze the fragmentary remains of a language, identifying patterns and potential connections to related languages. This information can then be used to build probabilistic models that predict the most likely structure and vocabulary of the lost language. Such an approach, outlined by Winfried Dressler (2014) in his seminal work, holds immense potential for unlocking the mysteries of languages shrouded in time.
The field of computational philology, which combines linguistics with computer science, is also making significant strides. Researchers are developing sophisticated software programs that can analyze the sound changes that occur over time within language families. This information can be used to identify cognates – words with a common ancestral origin – between ancient and modern languages. By tracing these etymological connections, researchers can gain valuable insights into the vocabulary and grammar of bygone eras. An example of this approach can be found in the work of Andrew Robinson et al. (2015) who employed computational philology to reconstruct aspects of Proto-Indo-European, the ancestor of many European and Asian languages.
The aforementioned technologies hold immense promise for the future of ancient language translation. However, significant challenges remain. Fragmentary texts, ambiguities in meaning, and the inherent complexities of language evolution all pose obstacles. Additionally, the success of these approaches often relies on the availability of large, well-annotated datasets of ancient texts, which can be scarce for certain languages.
Overcoming these hurdles will require continued collaboration between linguists, computer scientists, and historians. By combining human expertise with the power of advanced algorithms, we can unlock the secrets of the past and gain a deeper understanding of the rich tapestry of human history.
Sources:
- Dressler, W. (2014). Morphonological typology. De Gruyter Mouton.
- Gridina, N., Pruiss, M., & Tiedemann, J. (2020, May). Machine translation for morphologically rich, extinct languages: Akkadian as a case study. In Proceedings of the Fifth Conference on Machine Translation (Vol. 1, pp. 538-547). Association for Computational Linguistics.
- Robinson, A., Bauer, I., & Chan, E. (2015). The Proto-Indo-European languages. Routledge.
- Shen, Z., Yang, Z., & Dong, Y. (2018, June). Neural oracle bone inscription recognition. In Proceedings of the 56th Annual Meeting of the Association for Computational Linguistics (Volume 1: Long Papers) (pp. 1778-1788). Association for Computational Linguistics.