Ethical AI: Where Technological Advancement Meets Moral Responsibility
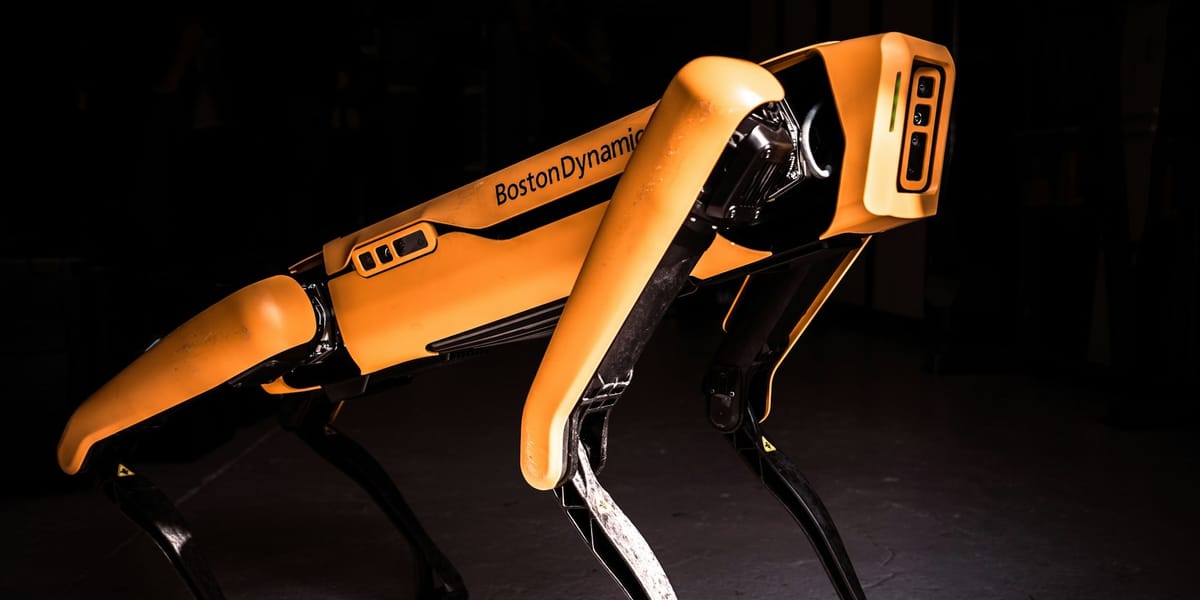
Artificial Intelligence (AI) has permeated countless aspects of modern life, from social media algorithms to self-driving cars. While AI promises extraordinary benefits, it also raises profound ethical concerns. The field of Ethical AI seeks to guide the development and use of these technologies to ensure they serve the common good and uphold principles of fairness, transparency, and accountability.
The Need for Ethical AI
Unquestionably, AI brings immense potential. It can help solve complex problems, streamline processes, and improve healthcare outcomes. However, if left unchecked, AI systems can perpetuate biases, threaten privacy, and even facilitate harmful decision-making. Some of the core ethical challenges surrounding AI include:
- Bias: AI algorithms trained on biased datasets may produce discriminatory results. For example, facial recognition systems have been shown to have higher error rates for people of color, highlighting how underlying biases can amplify existing social inequalities.
- Lack of Transparency: Many AI systems, especially those using deep learning, are "black boxes." Developers themselves may not fully understand how these systems arrive at their decisions. This lack of transparency makes it difficult to identify and address potential biases or unintended consequences.
- Privacy Concerns: AI often relies on massive amounts of personal data, raising concerns about how this data is collected, used, and protected. Data breaches or misuse can have serious privacy implications.
- Accountability: When AI systems make consequential decisions, especially in sensitive areas like healthcare or employment, who is responsible for harmful outcomes? The complex interplay between human developers and autonomous systems makes assigning accountability a challenging task.
Guiding Principles for Ethical AI
In response to these challenges, researchers and practitioners are working to establish frameworks for Ethical AI. These frameworks generally revolve around core principles such as:
- Fairness: AI systems should strive for equitable outcomes, avoiding discrimination based on factors like race, gender, or socioeconomic status.
- Transparency: Developers must promote explainability, allowing users to understand how AI systems reach their decisions. This is vital for trust and for identifying potential biases.
- Privacy: The collection and use of personal data must adhere to strict privacy standards and prioritize user autonomy.
- Accountability: Clear lines of responsibility must exist for AI systems, both during development and deployment.
Translating Principles into Practice
The development of Ethical AI is an ongoing effort. Here are some key strategies gaining traction:
- Bias Mitigation: Researchers are developing techniques to identify and mitigate biases within both training data and algorithms themselves.
- Explainable AI (XAI): Development of tools and techniques that offer insights into the reasoning of AI systems, helping identify potential issues and facilitating greater trust.
- Algorithmic Auditing: Third-party assessments of AI systems to evaluate their adherence to ethical principles.
- Policy and Regulation: Growing calls for governmental regulations and industry standards governing the collection of data and the development and use of AI systems.
Conclusion
Ethical AI is not merely about limiting risks; it's about harnessing this transformative technology responsibly and equitably. As AI continues its rapid evolution, collaboration among technologists, ethicists, policymakers, and the public is crucial to align AI's potential with human values.
Research Sources
- Floridi, L., & Cowls, J. (2019). A unified framework of five principles for AI in society. Harvard Data Science Review, 1(1).
- Hagendorff, T. (2020). The ethics of AI ethics: An evaluation of guidelines. Minds and Machines, 30(1), 99-120.
- Jobin, A., Ienca, M., & Vayena, E. (2019). The global landscape of AI ethics guidelines. Nature Machine Intelligence, 1(9), 389-399.